Valeo.ai
We are building an artificial intelligence research center for automotive applications based in the center of Paris, a project started in 2017 to conduct ambitious research projects, regarding assisted and autonomous driving
Artificial intelligence is already at the core of the transformation of the automotive industry! 10 years ago, there was no real AI in cars. Today, most are packed with software, part of which is AI related. Artificial intelligence is changing mobility everywhere!
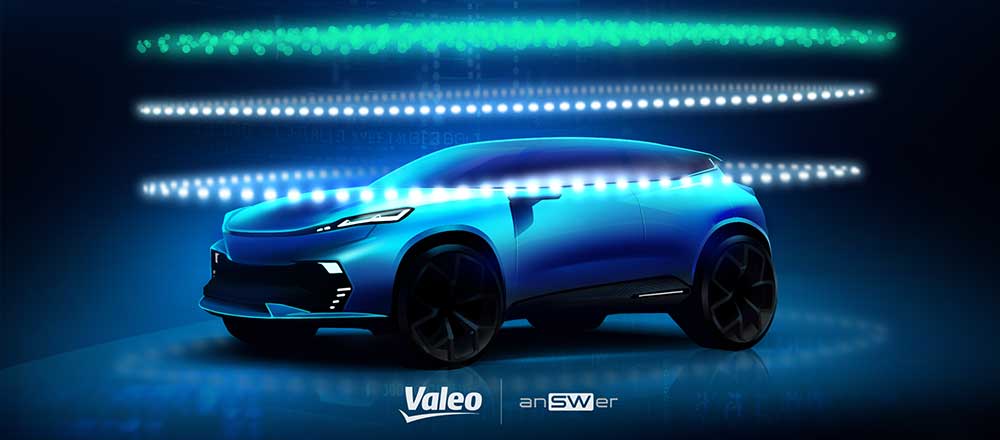
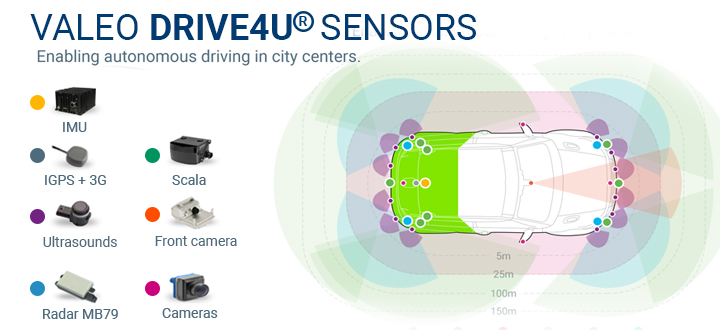
Multi-sensor perception
Automated driving relies first on a diverse range of sensors, like Valeo’s cameras, LiDARs, radars and ultrasonics. Exploiting at best the outputs of each of these sensors at any instant is fundamental to understand the complex environment of the vehicle. To this end, we explore various deep learning approaches where sensors are considered both in isolation and collectively.
Domain adaptation
Deep learning and reinforcement learning are key technologies for autonomous driving. One of the challenges they face is to adapt to conditions which differ from those met during training. To improve systems’ performance in such situations, we explore so-called domain adaption techniques, as in AdvEnt, our project presented at CVPR 2019.
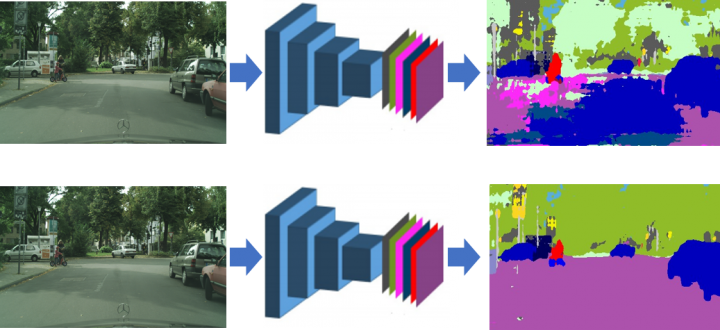
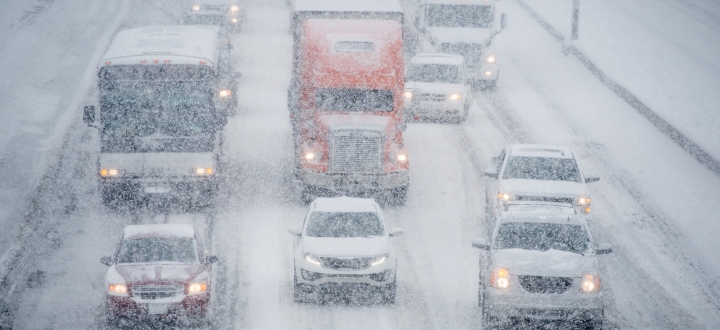
Uncertainty estimation
When the unexpected happens, when the weather badly degrades, when a sensor gets blocked, the embarked perception system should diagnose the situation and react accordingly, e.g, by calling an alternative system or the human driver. With this in mind, we investigate automatic ways to assess the uncertainty of a system and to predict its performance.
Meet our team
-
Ph.D. student Florent Bartoccioni
Ph.D. student
Perception | Scene understanding | Dynamic forecasting
ENS Rennes | CTU Prague | INRIA
-
Research Scientist Alexandre Boulch
-
Research Scientist Andrei Bursuc
-
Research Scientist Mickaël Chen
-
Principal scientist Matthieu Cord
-
Research Scientist Spyros Gidaris
-
Research Scientist David Hurych
-
Principal scientist Renaud Marlet
-
Research Scientist Gilles Puy
-
Research Scientist Oriane Siméoni
-
Research scientist Tuan-Hung Vu
-
Research Scientist Eloi Zablocki
-
Ph.D. student Léon Zheng
Ph.D. student
Machine Learning | Frugal Learning
X | MVA | ENS Lyon
Recent Activities
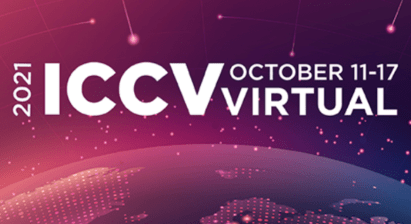
Valeo.ai x ICCV’21
Valeo.ai participates to ICCV, the premier computer vision conference, in October 2021, presenting six papers on the challenge of understanding complex scenes with cameras, radar or lidar.
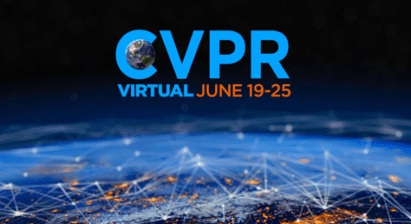
Valeo.ai x CVPR’21
Valeo.ai participates to CVPR , the premier computer vision conference, in June 2021, presenting three papers, contributing to a tutorial on self-supervised learning, co-organizing the workshop on omnidirectional computer vision and presenting keynotes to SafeAI4AutonomouDriving and Vision4AllSeason workshops.
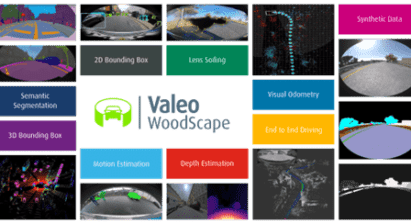
Woodscape dataset
Four Valeo teams collaborate to release Woodscape, the first public multi-sensor driving dataset with fisheye cameras, named after Robert Wood who invented the fisheye camera in 1906, featuring 9 perception tasks such as 2D and 3D object detection, semantic segmentation and depth estimation.
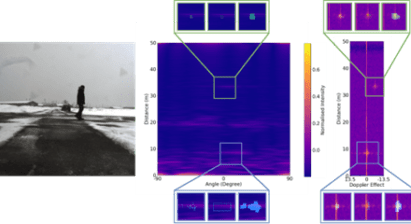
Carrada dataset
In collaboration with researchers at Télécom Paris, Valeo.ai releases the Camera and Automotive Radar with Range-Angle-Doppler Annotations (Carrada) dataset, the first public automotive radar dataset with cars, cyclists and pedestrians precisely annotated in the raw signals.
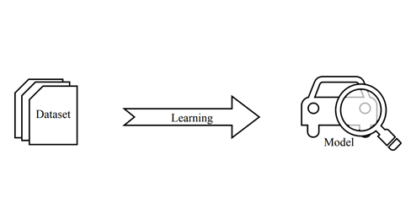
Can we make driving systems explainable?
Valeo.ai researchers release a comprehensive survey on the explainability of vision-based driving systems, presenting a wide range of existing techniques for post-hoc or by-design explainability, analysing their current limitation and outlining future research avenues toward a better interpretation of self-driving AI models.
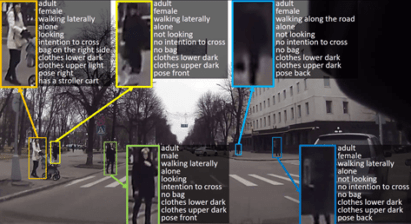
Paying attention to vulnerable road users
Vulnerable road users such as pedestrians must be reliably analysed by ADAS and AD systems; Valeo.ai shows that synthetic people in real scenes help to train better detectors (collaboration with CTU Prague) and that a multi-task model can recognize up to 32 attributes, including action and attention (collaboration with EPFL).